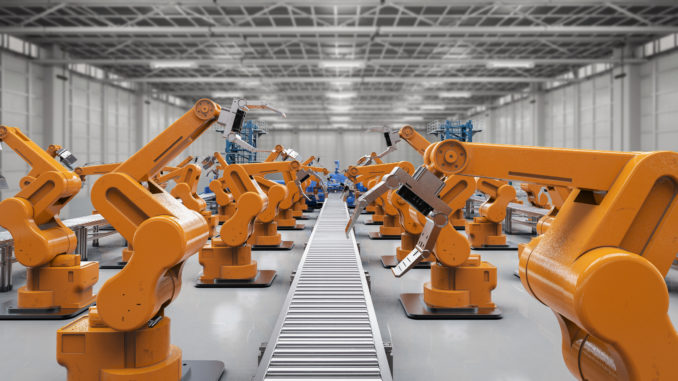
This week, we continue a special series of blogs on the future of economic development, work, and wages in developing countries. We look at employment effects of automation in developing countries.
A tricky empirical question
At first sight, the case looks simple: surely, automation will reduce employment by making certain forms of (routine) work superfluous?
But what if, in a competitive market, productivity gains thanks to automation lead to lower prices and thus higher quantities demanded? Could the net job effects be positive? Could the demand for new labour-intensive tasks rise as the cost of labour falls relative to capital? These are empirical questions and empirical studies are thus needed to evaluate if and in what sectors automation de facto reduces labour demand.
So, what is the scholarly verdict? Alas, it’s complicated. Empirical estimates and forecasts of the potential impact of automation across the world range from alarming scenarios, according to which there is a “50% chance of AI outperforming humans in all tasks within 45 years” (Grace et al.), on the one hand, to contrasting claims of there being “no evidence that automation leads to joblessness” (Mishel & Bivens), and the sarcastic recommendation that “everyone should take a deep breath” (Atkinson & Wu).
The seminal study in the recent automation literature is that of Frey and Osborne for the US, and subsequent studies have reproduced and refined their methodology. They conclude that almost half of the US employment is ‘at risk’. In contrast, Arntz et al. occupy a middle ground in terms of optimism. The authors argue with some plausibility for a ‘task-based’ rather than an—inevitably over-simplified—’occupation-based’ approach to estimating automatability risk. Arntz et al. draw on data from an international survey of adult skills conducted across OECD countries which contains data on the tasks performed for each type of job. The authors use these data to impute a score of automatability, as well as the size of the population at ‘high risk’ of automation. Interestingly, Russia’s occupational structure is deemed least automatable of the 21 countries considered, whereas Germany and Austria top the rank. Put differently, the country with the lowest gross domestic product (GDP) per capita (and per worker) in the data set considered by Arntz et al. shows the highest resilience to automation. Generally, there is no consistent relationship with GDP per capita and their score of automatability, in this OECD data set (which is thus based on a selection of structurally fairly similar economies).
The World Bank, based on a similar methodology, estimates 1.8 billion jobs or two-thirds of the current labour force of developing countries are estimated to be susceptible to automation from today’s technological standpoint. Their data, which, while patchy do go substantially beyond OECD countries, show a clear relationship with economic development – and are thus revealing regarding the implications of technological innovation for developing countries.
What does this mean for the future of structural economic transformation?
We can say, assuming these automatability estimates are reasonable, that the labour force of more service sector-based, richer economies tends to be much less replaceable compared to more agriculture-based, poorer economies. This pattern is intuitive and is explained by the complexity and creativity of service-sector work and the amount of face-to-face human interaction involved in it. If we break down the relationship of sectoral employment by level of GNI per capita, the above-mentioned pattern largely holds. Among HICs, there is no relationship between agriculture and automatability simply because there is almost no employment in agriculture. Industrial work is more automatable and service-sector work less automatable across both country groupings, so the level of economic development does not moderate that sectoral relationship.
If countries have to decide how to reallocate employment during structural change and the described cross-country pattern allows any inference about country-level developments over time, an increase in service-sector employment would suggest itself as the only future-proof employment growth model. In HICs, it would suggest structural change away from industrial work and in developing countries away from agriculture.
Holding all else constant, sectoral differences in the replaceability of labour will sustain a pressure for both further deindustrialization and deagriculturalization. This is not a new phenomenon: in fact, the cross-country pattern of sectoral employment shares shows a surprising degree of continuity over time. What appears to be happening, though, is an expansion of service-sector employment in the richest countries, and a reduction in the share of industrial work compared to the early nineties (this pattern is corroborated by Wood). In line with this, Chandy speculates that “China may be one of the last countries to ride the wave of industrialization to prosperity”.
Generally, most of the global cross-country variability of employment shares is found toward the low end of the GNI per capita, whereas countries above a per capita GNI of 20,000 look structurally very similar, i.e. are highly service-based and thus face lower automatability. In general, it is only in the poorest countries that a considerable proportion of labour is in agriculture. However, even in middle income developing countries such as Indonesia and Thailand, a third of the labour force remains in agriculture—an astonishing persistence of arguably highly automatable work.
Agriculture employs only a few per cent of the labour force in wealthy countries. This suggests that in contrast to OECD countries, many jobs in developing countries have likely been automatable for a long time. In the past, industrialised countries have managed to achieve vast agricultural surpluses with minimal labour input. Fueled by agricultural subsidies, these surpluses have flooded world markets with cheap produce. Developing country producers, beware: industrial robotisation promises a similar scenario with manufactured goods.
We’ll continue the series of blogs with some discussion next of public policy responses to automation.
Lukas Schlogl is a Research Associate with the ESRC GPID Research Network at King’s College London. He works on structural change, digital transformation, and political behavior in developing countries.
Andy Sumner is a Reader in International Development in the Department of International Development, King’s College London. He is Director of the ESRC Global Poverty & Inequality Dynamics (GPID) Research Network.