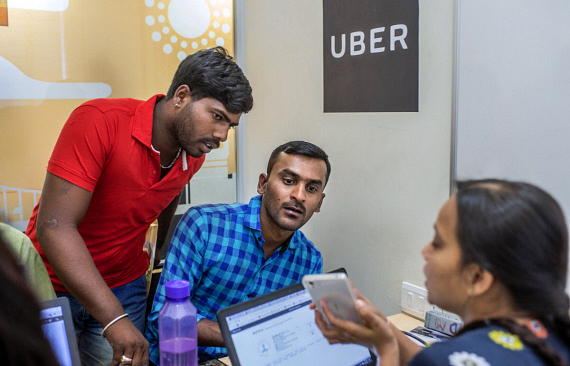
This week, ESRC GPID Director, Andy Sumner continues continues the set of blogs on deindustrialisation and developing countries.
In past weeks, we’ve discussed varieties of deindustrialisation and drivers. This week, we look at the relationship between deindustrialisation and inequality.
So, what are the distributional consequences of deindustrialisation in the developing world?
First, a recap – why focus on deindustrialisation? Many middle-income developing countries are not experiencing the ‘traditional’ model of economic development and structural transformation of industrialisation, but instead, a deindustrialisation or tertiarisation process, having built a manufacturing base which has faltered as China and other competitors have entered global value chains. Even in the very poorest countries, without a manufacturing base, the shift has not been from agriculture to manufacturing, but from agriculture to services.
Many books have been written on these processes of deindustrialisation and tertiarisation in the advanced countries, dating back to the 1980s and focusing on the US and UK or other OECD countries. There are also a set of seminal papers on the inverted U curve for manufacturing. However, the comparative study of deindustrialisation in developing countries has received relatively limited attention to date, other than in some key papers by Palma and Rodrik and also Treganna.
There has, however, been much attention paid to deindustrialisation in a set of policy reports of the UN and other international agencies. A range of international agencies have also drawn attention to issues relating to deindustrialisation such as the United Nations Industrial Development Organization (UNIDO), the World Bank and others.
Indeed, it was the United Nations Conference on Trade and Development (UNCTAD) who introduced the concept of ‘premature deindustrialisation’, the first component of which is that ‘peak manufacturing’, in employment or gross domestic product (GDP) shares (one could add export shares), has been reached and the inverted U curve is now on the plateau or even downswing of the curve.
The second component is that, with reference to developing countries versus developed countries, the inverted U curve has moved leftwards over time. This means that the point at which the inverted U turns is, on average, lower in per capita income terms now than in the 1990s, which was already lower than in the 1980s. Also, the inverted U curve has shifted downwards, meaning that the peak manufacturing shares of developing countries are lower than those of advanced countries.
Whether ‘premature’ or not, deindustrialisation in developing countries raises a set of new questions, to date little explored.
One of those questions is: ‘What are the distributional consequences of deindustrialisation in the developing world?’
If one takes a look at the latest edition of the World Bank’s World Development Indicators data set, and takes a definition of five years or more for episodes of deindustrialisation, we observe three things: first, we find 46 country episodes of industry value-added shares contracting in developing countries. Second, we find – not surprisingly – that manufacturing and industry shares almost always tend to contract together. Third, we find that it is services that expand in most cases but – surprisingly – in a fifth of cases, it is agriculture that expands.
We also find 50 episodes where employment shares in industry contracted. Again, in most cases, services expand shares, but as before, in a fifth of cases, agriculture shares expanded.
What can be said about the inequality impact of these movements? Of course, governments can and do intervene to manage inequality as we have discussed with reference to South East Asia.
That said, the underlying dynamics unleashed by different patterns of structural change and deindustrialisaton in particular tell us what public policy has to deal with.
Deindustrialisation and inequality dynamics
What happens to income inequality if labour moves from the manufacturing sector to the service sector, which is dominated by low value-added and informal sector jobs?
The distribution dynamics of industrialisation were of central concern to Kuznets and his seminal work is well known. In that seminal work, he hypothesised that inequality would rise during industrialisation as labour moves from the more equal sector to the less equal sector. What if labour moves from manufacturing to services?
Kuznets argued that income inequality in a dual sector economy is an aggregation of (i) income inequality in each sector; (ii) the mean income of each sector; and (iii) the population shares in each sector. Thus, even the population shift itself could raise inequality, as Kuznets himself noted.
The movement of workers itself towards a sector with lower value-added/informal service jobs will drive down wages in itself. This will hinder poverty reduction and likely put upward pressure on national inequality, weakening the poverty-reducing power of economic growth, and potentially placing the existing social contract under strain, or even possibly limiting the emergence of more inclusive social contracts. Deindustrialisation also means a weakening of the traditional working class in manufacturing, which may curtail political pressure on elites for more equitable growth.
Although the Kuznets hypothesis is dismissed as a universal law, rising inequality in some fast-growing developing countries has renewed interest in distributional dynamics during economic development. There have been various attempts at new theory-building on how income inequality evolves with economic development in the Kuznetsian tradition. For example, Galbraith argues that it is global forces that have driven the changes in national inequality since 1970. The key drivers of the changes in national inequality are world interest rates and commodity prices (and between-sector terms of trade). Lindert and Williamson argue that it is the shift towards market orientation (domestic to export) of agriculture and not the shift from agriculture to manufacturing and services that causes inequality to rise.
Roine and Waldenström suggest a new Kuznets curve based on technological developments starting, not a sectoral shift of agriculture to industry, but a shift from traditional industry to technologically intensive industry based on Tinbergen’s hypothesis on the race between education and technology, and returns to education. Oyvat, argues that it is agrarian structures – national land inequality – that are deterministic. Finally, Acemoglu and Robinson discuss the Kuznets curve with a focus on political economy and two models of late capitalism. They argue that when the process of industrialisation does increase inequality, this leads to the political mobilisation of the masses that are concentrated in urban areas and factories. Political elites thus undertake reform to ensure their continued position at the top.
None of these new theories in the Kuznetsian tradition consider a different form of structural transformation – deindustrialisation. Given that many developing countries are experiencing, not an industrialisation process, but a deindustrialisation process, what are the distributional dynamics of this process and how do they differ to industrialisation?
If we look again at the World Bank’s World Development Indicators, and the UNU-WIDER Standardised WIID database for income inequality (Baymul and Shorrocks, forthcoming), we find some interesting associations (without making any claims to causation on that alone), and we can tentatively explore associations with changes in the net (post tax and transfers) income Gini. To reiterate, this is not to make claims for causality but to create further empirical insights.
What do we find? The net Gini is negatively correlated with industry employment, which means that inequality rose as industry sector shares contracted. We find that the net Gini is correlated positively with agriculture employment shares, which means inequality falls as agriculture employment shares rise.
In terms of value added, we find that the net Gini on average rose when manufacturing or agriculture shares contracted. The net Gini is negatively correlated with manufacturing GDP shares and agriculture GDP shares, which means that inequality rose when the size of these sectors fell. The Gini on average fell when shares on non-manufacturing industry and services fell. The net Gini is correlated positively with non-manufacturing industry GDP shares and service sector GDP shares, which means inequality rose as these sectors expanded.
If we instead use the Groningen Growth and Development Centre’s 10-Sector dataset, we can consider a smaller set of developing countries and 20 deindustrialisation episodes of five years or more. The associations are the same as above. We find that, on average, the expansion of manufacturing or agriculture is associated with a falling net Gini. The net Gini is negatively correlated with manufacturing shares (GDP and employment) and agriculture (GDP and employment) and construction GDP shares. The net Gini is positively correlated with mining shares (GDP and employment), shares of financial intermediation, renting and business activities (GDP and employment), government (GDP and employment shares), other shares (GDP and employment), and wholesale, retail and trade employment shares. To reiterate, these are correlations.
In sum, the association suggests that there is an unequalising effect of deindustrialisaton if there is a movement towards services, and an equalising effect if the movement is towards agriculture. The fact that deindustrialisation is associated with different distribution outcomes points again towards varieties of deindustrialisation and differing distribution dynamics.
Next week, we discuss a new GPID working paper by Sen and Baymul who explore such issues with far greater sophistication than the outline above, and present interesting results on different forms of structural transformation and their inequality dynamics.
Andy Sumner is a Reader in International Development in the Department of International Development, King’s College London. He is Director of the ESRC Global Poverty & Inequality Dynamics (GPID) Research Network.